SCHOOL | 3-4-5 June 2024
Data Science in Fundamental Physics
Indico Link to talks & materials
SPEAKERS
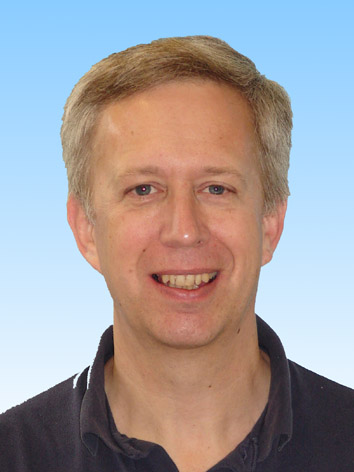
Glen Cowan
Professor of Physics at Royal Holloway, University of London. Coordinator and developer of statistical methods in ATLAS experiment at CERN.
Author of the book “Statistical Data Analysis”. His current research is focused on analyzing data currently being collected by the ATLAS Experiment. Specifically, he is involved in coordinating and developing statistical methods and software for use by many studies carried out with ATLAS data.
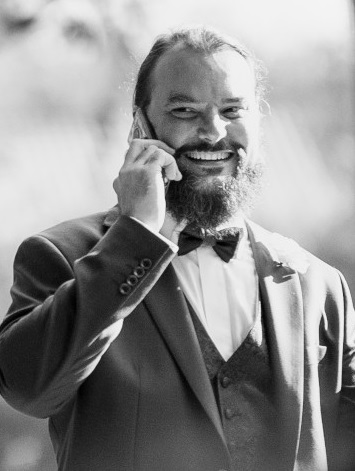
Pietro Vischia
Ramón y Cajal senior researcher at the Universidad de Oviedo and ICTEA.
Machine Learning coordinador of the CMS Experiment at CERN.
Specialist in Machine Learning applied to High Energy Physics. Researcher in high-dimensional spaces via gradient descent, eventually powered by quantum algorithms, and on the extension of machine learning methods to realistic neurons with spiking networks, to be then implemented in neuromorphic hardware devices.
Assistants: Lidia Carcedo, Imanol Corredoira, Carlos Hervés, Miguel Martins, Martín Pérez Maneiro, Felix Riehn, Emilio Xosé Rodríguez, Sara Sellam
TIME
9:30 – 11:15
11:15 – 11:45
11:45 – 13:30
13:30 – 14:00
14:00 – 15:30
15:30 – 17:00
17:00 – 17:30
17:30 – 19:00
Monday 3
Tuesday 4
Wednesday 5
Probability & Statistics – Glen Cowan (15 minutes break included)
Coffee break
Machine Learning – Pietro Vischia (15 minutes break included)
Buffer time | 8 min walk to the lunch venue (Auditorio de Galicia)
Lunch
Hands on!
Coffee break
Hands on!
Probability & Statistics – Glen Cowan
1st Lecture
Probability, Bayes vs. Frequentist | Frequentist parameter estimation | Hypothesis tests
2nd Lecture
p-values | Confidence limits | Systematic uncertainties | Bayesian parameter estimation
3rd Lecture
Significance, sensitivity | Bayes factors | Models for anomalies
Machine Learning – Pietro Vischia
1st Lecture
Mathematical foundations of ML | Vapnik’s theory of statistical learning | Early methods from statistics to ML: PCA, SVM, decision trees
2nd Lecture
Supervised learning: neural networks, gradient descent | Technical foundations: automatic differentiation | Hardware foundations: from CPUs to GPUs, TPUs, FPGAs, ASIC, neuromorphic circuits | Practical techniques (e.g. hyperparameters optimization, regularization)
3rd Lecture
Transformers, large (language models) | Spiking networks | Unsupervised learning | Quantum machine learning